In the driving seat: An interview with Cancer Mutation Census’s Senior Bioinformatician, Bhavana Harsha
25 Nov 2021
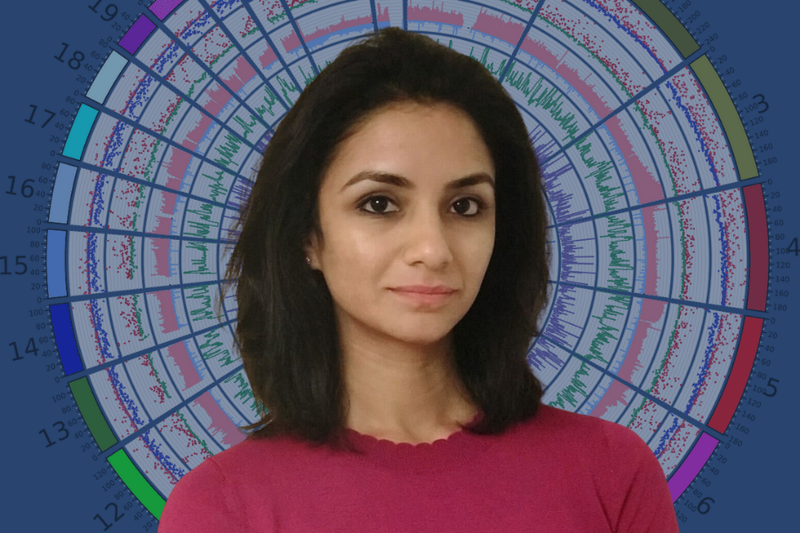
Q1. Can you tell me a little bit about your background and how you ended up at COSMIC?
I have an undergraduate degree in Biotechnology and a Master’s in Bioinformatics. Back in 2016, I saw an advert for a Senior Bioinformatician at COSMIC. It got me looking into the work they do which I thought was interesting and important. I applied and got the job. It's been amazing and I’ve never looked back since.
Q2. And you work primarily on the Cancer Mutation Census (CMC). Can you describe it in one sentence?
I would say that CMC is an attempt to identify and characterise the somatic mutations driving cancer.
Q3. That was impressively succinct! To a bit more depth, how did you develop CMC?
The CMC was developed at COSMIC by Zbyslaw Sondka, our Head of Science, Celestino Creatore and me. We’ve worked on a system that categorises mutations seen in cancer into Tiers based on their importance. This classification is based on evidence we have within COSMIC as well as evidence from resources like ClinVar and algorithms like dNdScv, which calculates dN/dS scores.
Q4. You’ve just mentioned ClinVar – what is this and what is its relationship to COSMIC CMC?
ClinVar is a resource where users submit variant data from patient samples from all diseases. For CMC, we take the subset of ClinVar, which has data on cancer variants. We’re interested in the variants, which are classified as ‘Pathogenic’ or Likely Pathogenic’. These act as added evidence for variants that we have in COSMIC. If it’s also been noted in ClinVar and been assessed as pathogenic then it adds weighting to the evidence that the variant is causing the cancer.
Q5. Okay, perfect. And then on to dN/dS ratios. Why are these important?
A dN/dS ratio is the ratio of non-synonymous to synonymous mutations in a cell. Traditionally, this is used in species evolution but the same principle was applied by our colleagues from the Sanger institute to study cancer cells. Developed by Inigo Martincorena’s team, this algorithm calculates the global rate of mutation compares it to each single site to see just how much it has been mutated.
A dN/dS ratio of one means there's a neutral rate of mutations within the cell. Mutations beneficial for cancer cells i.e. driving the disease, are expected to be seen more frequently in cancer population than if they occurred randomly. Such mutations can be identified by the dN/dS ratio higher than one. In the context of somatic evolution, this tells us that certain mutations present a selective advantage because they bring about survival and proliferation of the cancer cell.
This way, we’re able to look in more depth at specific mutation sites and if there’s a high dN/dS ratio at a particular site, we can say, ‘yes, it’s likely this site is under positive selection for that cancer cell and this mutation is helping this cell survive’.
We use this within the CMC as another set of evidence for driver mutations.
Q6. As you already said, CMC identifies likely driver mutations in cancer, in case there’s any ambiguity, what exactly do you mean by driver mutation?
To put it simply, a driver mutation is a genomic alteration that is shown to cause cancer.
And to explore it more in detail. There are cancer driver genes that are already well-known, and this is the work of decades of cancer research. These are certain genes, that when mutated, enable oncogenic transformation. However, an immense load of mutations is a hallmark of many cancers. Most of these mutations occur as a result of processes driving the disease and do nothing to accelerate it. Only very small fraction of mutations within cancer-driving genes have the actual capacity to give them oncogenic properties. Our aim is to identify these mutations.
We look at collective evidence within COSMIC and other sources to identify mutations that are seen repeatedly and have a high sample recurrence within cancer patients. We then combine it with other parameters like germline population frequencies to rule out common non-causative variants.
After running in-depth algorithms, we take the set of mutations and classify them into three tiers. Tier-one mutations are the most significant and most likely to be driving the cancer.
Although CMC is only focusing on coding mutations currently, it's a first step towards identifying a set of mutations that we believe are good candidates for driving mutations, and it’s backed by a wealth of evidence.
Q7. And speaking to how comprehensive CMC is. Currently, CMC only identifies coding mutations. But are there cases where non-coding mutations are drivers for cancer?
Yes, absolutely. There's a lot of research being done to investigate the role of non-coding mutations within cancer. And we have a lot of data within COSMIC about non-coding mutations, coming in from whole genome screens. It would be interesting to explore this in more detail, as there's tremendous insight to be found from non-coding mutations in cancer.
Q8. To take a quick step back, you spoke about population frequencies just now – can you elaborate?
We want to identify the harmless variants that are seen in healthy individuals. We can do this by incorporating data about variant allele frequencies from the genome aggregation database (gnomAD), within datasets which were classified as controls in disease case-control studies that have been conducted globally. This is what we mean by normal or healthy population.
Q9. What’s unique about CMC?
It’s the way we integrate manually curated annotations (including data from the Cancer Gene Census) with COSMIC data on frequency and data from dN/dS scores. As far as I'm aware, this is the only resource that is using various types of cancer genomics data to collate driver mutations.
As to what else makes us unique? It’s our transparency. We provide tier one, two, and three mutations and we also present all the supporting evidence. This means if people wanted to make their own inferences about what was interesting, tweak the scoring system, run it on their own data etc. then they're free to do this. It means our users can come up with their own set of interesting mutations.
Q10. You’ve just mentioned one way people could manipulate the data, which leads me onto the next and final question about CMC, how can people use CMC? What will the data be useful for?
It’s useful as a resource detailing the mutations most likely driving cancer and would make excellent candidates for drug discovery. So there’s an obvious use in the Pharmaceutical industry.
And similarly, for diagnostic purposes. Imagine being able to sequence a patient, identify the driver mutations through comparison with CMC, and then combine this with other COSMIC data like Actionability and Drug Resistance. This insight will drive precision oncology.
You can learn more about COSMIC CMC on this page.